Treating gaps and biases in biodiversity data as a missing data problem
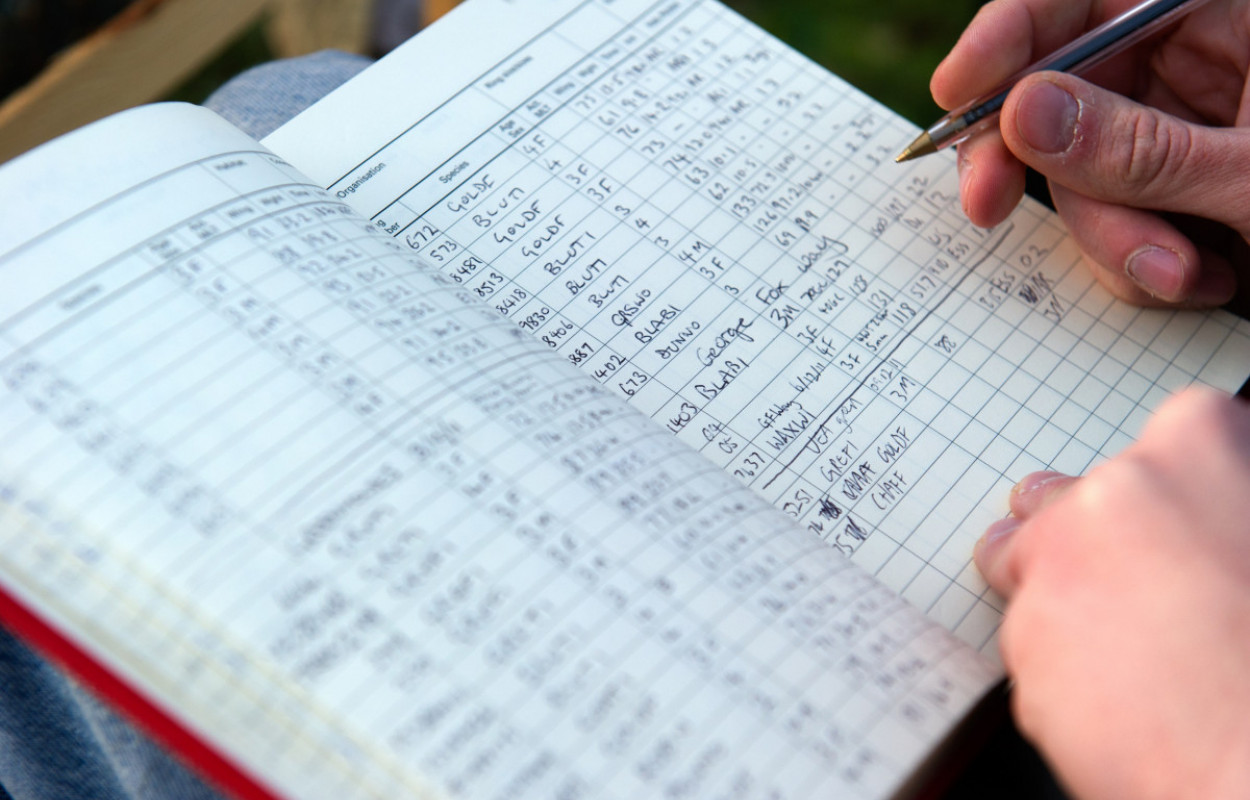
Author(s): Bowler, D.E., Boyd, R.J., Callaghan, C.T., Robinson, R.A., Isaac, N.J.B. & Pocock, M.J.O.
Published: August 2024
Journal: Biological Reviews
Digital Identifier No. (DOI): 10.1111/brv.13127
The value of data collected by volunteers is inestimable and they have been used in myriad ways to address many pressing conservation problems. One big benefit is that much more data can be collected than could ever be managed if only paid staff were relied upon. This means that the data gathered can cover much larger areas and be more representative of the country as a whole.
However, such data do need to be treated carefully if they are to yield reliable evidence. One problem that frequently occurs is that of gaps in coverage caused by, for example, missing visits. In any survey, some visits will be missed for a variety of good reasons: the surveyor was away on holiday, the weather was too bad, or there was an issue with site access, among others. The question is, do these missing visits matter? Will they alter the conclusions we might draw from the data?
A branch of mathematics deals with something called sampling theory that considers questions like how many squares do you need to sample, and where, to detect a particular level of change in something, given that it is rarely possible to count in every square. They have considered this problem of missing data in some detail. Whether or not it matters depends on the pattern of the ‘missingness’ – is it essentially random (with respect to the survey), the surveyor taking a holiday for example, or not random, for example birds may flock together more in cold weather, so missing a visit because it is too cold or icy to go out might miss this.
This paper reviews the work that has been done in this arena and evaluates the range of strategies available to deal with it. The best approach, inevitably, depends on the precise question being asked – are we trying to work out how many birds there are (population size), or simply whether more birds are found in certain habitats or associated with particular features? While formal consideration of such issues might seem a million miles from watching birds in a field or windswept estuary, it can be very helpful when it comes to making the most of the data being collected. The paper then provides some recommendations on how best to deal with missing data to help improve survey design and analysis to ensure volunteer-collected data remain relevant in the face of the continuing biodiversity crisis.
Abstract
Big biodiversity data sets have great potential for monitoring and research because of their large taxonomic, geographic and temporal scope. Such data sets have become especially important for assessing temporal changes in species’ populations and distributions. Gaps in the available data, especially spatial and temporal gaps, often mean that the data are not representative of the target population. This hinders drawing large-scale inferences, such as about species’ trends, and may lead to misplaced conservation action. Here, we conceptualise gaps in biodiversity monitoring data as a missing data problem, which provides a unifying framework for the challenges and potential solutions across different types of biodiversity data sets. We characterise the typical types of data gaps as different classes of missing data and then use missing data theory to explore the implications for questions about species’ trends and factors affecting occurrences/abundances. By using this framework, we show that bias due to data gaps can arise when the factors affecting sampling and/or data availability overlap with those affecting species. But a data set per se is not biased. The outcome depends on the ecological question and statistical approach, which determine choices around which sources of variation are taken into account. We argue that typical approaches to long-term species trend modelling using monitoring data are especially susceptible to data gaps since such models do not tend to account for the factors driving missingness. To identify general solutions to this problem, we review empirical studies and use simulation studies to compare some of the most frequently employed approaches to deal with data gaps, including subsampling, weighting and imputation. All these methods have the potential to reduce bias but may come at the cost of increased uncertainty of parameter estimates. Weighting techniques are arguably the least used so far in ecology and have the potential to reduce both the bias and variance of parameter estimates. Regardless of the method, the ability to reduce bias critically depends on knowledge of, and the availability of data on, the factors creating data gaps. We use this review to outline the necessary considerations when dealing with data gaps
at different stages of the data collection and analysis workflow.
Share this page